Meta Learning
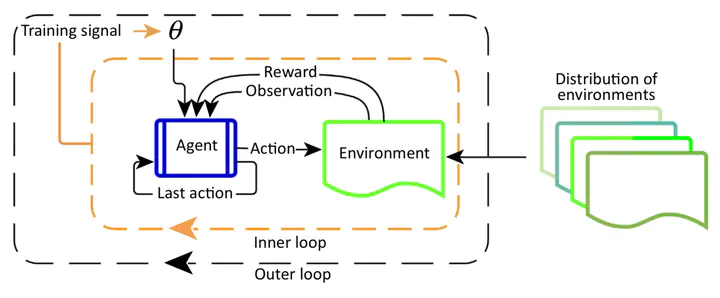
Sample efficiency is key to the applications of many real-world AI for science tasks, e.g., drug discovery.
Recently, I realize that the inner-loop in the meta-learning framework is the key bottleneck preventing it from being deployed. I’m exploring ways to create more forms of inner-loop APIs.
Our Works
- Massive Editing for Large Language Models via Meta Learning, ICLR 2024
- Think Before You Act: Decision Transformers with Internal Working Memory, arXiv 2023
- Learning Multi-Objective Curricula for Robotic Policy Learning, CoRL 2022
- Bidirectional Learning for Offline Infinite-width Model-based Optimization, NeurIPS 2022
- Evolving Decomposed Plasticity Rules for Information-Bottlenecked Meta-Learning, TMLR 2022